Download our latest report - Protecting & growing your payments business -
here Opens in a new window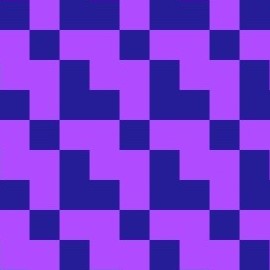
The Thredd team
April 23, 2025
Fraud detection systems that can learn and adapt without human intervention using machine learning have become a key weapon in the battle against fraudulent payments.
The Thredd team
For over a decade, artificial intelligence (AI) has been touted as the solution to society’s biggest challenges – from combatting climate change and fighting disease through to increasing workforce productivity.
But it is only in the last couple of years that the transformative potential of AI has started to be realised in broader applications.
Problems thought to be decades away from a solution – like cataloguing structure predictions for nearly every protein known to science – are now being completed with unprecedented speed and accuracy through solutions such as the Alphafold project. And since ChatGPT emerged in November 2022, placing generative AI centre stage in the hands of 100m consumers in two months, the pace and scope of innovation has been dizzying.
Generative AI is starting to revolutionise nearly every industry: designing buildings, forecasting natural disasters, automating code writing, enhancing financial market predictions, and helping driverless cars make the right decisions in real-time – among many other use cases.
And within the payments industry, while early forms of AI have been aiding back-office operations for many years, the implementation of generative machine learning is a game changer for fraud detection, powering better intelligence, faster decisions, and greater accuracy.
The impact could be just as profound as it has been elsewhere. As Michael Jabbara, vice president and global head of fraud services at Visa, said in a recent interview, “[AI is] the superpower that gives us the ability to detect that proverbial fraudulent needle in the overall haystack of legitimate interactions, and then build the automation necessary to carve out the fraud while letting the authentic transactions go through.”1
Machine learning can be defined a sub-category of AI that gives computers the ability to learn without explicitly being programmed. Instead, they use algorithms and statistical models to automatically derive insights and recognise patterns from data.
In contrast to ‘traditional’ AI systems that require explicit instruction to classify data, generative AI models enable machines to learn patterns and structures within data and use that knowledge to generate relevant outputs autonomously.
There are two main approaches to machine learning: supervised and unsupervised. Under the supervised machine learning model, a data scientist teaches the algorithm what conclusions it should make by using a dataset with predefined outputs. Unsupervised machine learning uses data with no defined output, to train the computer to identify complex processes and patterns without human guidance.
The key characteristic of machine learning is that the technology produces increasingly accurate predictions over time – in the same way a human becomes more adept at a task with practice. This is particularly valuable where the data and the nature of the task are constantly changing.
The algorithms look for recurring themes and detect anomalies across vast amounts of data from multiple sources, creating data models that can make predictions around – for example – whether a payment is legitimate.
As Jim McCarthy, our CEO explains, machines didn’t become intelligent on their own. They require training based on huge amounts of data – and the quality of that data is the key to generating accurate results.
“The businesses with the best data models will be the winners,” he says. “Companies also really need to think about who they are going to partner with to build their AI systems. Payments are all about trust and push payments [where the payer takes the initiative to send money to the payee] are particularly vulnerable to fraud.”
There are several applications for machine learning in the payments industry, but fraud detection could be one of the most important.
It can be used in a number of scenarios, including anomaly detection – where the user sets pre-defined conditions that identify potentially fraudulent or erroneous payments – and risk scoring, where transactions are compared against predefined rules and past transactional data.
Other applications for machine learning include network analysis (examining connections between participants in the payments system), text analysis (mining of data contained in natural language), and identity verification.
The ability of machine learning to enhance these processes means it has huge potential benefits in areas from credit card, mobile and BNPL payments to loyalty programmes and online gaming.
And the value of machine learning should be considered in the context of the cost of global payment fraud. Almost half (46%) of the companies surveyed for PwC’s global economic crime and fraud survey 2022 reported experiencing fraud over the previous 24 months, while Juniper Research’s June 2023 online payment fraud report predicts that merchant losses from fraudulent online payments will exceed $362 billion between 2023 and 2028.
“Payment fraud has been around for several years, but it really spiked significantly since the Covid-19 lockdowns with authorised push payment fraud,” said Anthony Gudgeon, Head of Fraud Operations at Thredd. “Fraud is ever growing, ever changing, and a constant battle. But machine learning is helping us to fight back, with its ability to self-learn enabling us to better protect customers now and in the future.”
The challenge of combatting payment fraud is further heightened by fraudsters using machine learning themselves to make their scams appear legitimate.
No matter how skilled or experienced they may be, fraud prevention officers cannot hold back the tide without the help of dedicated technology.
In fact, Experian’s 2023 UK identity and fraud report describes machine learning as an essential component of any effective fraud strategy, while more than four-in-10 of the merchants surveyed for the 2023 global ecommerce payments & fraud survey were using machine learning to fine-tune fraud management.
The challenging landscape is one of the reasons why Thredd has partnered with Featurespace, a leading provider of adaptive behavioural analytics for fraud detection and risk management, to create an issuer processing fraud mitigation solution.
The machine learning aspect of this solution can interpret different metrics and characteristics to separate good data from bad, and is constantly learning and improving in a process known as adaptive learning.
This means that businesses don't have to commit resources to constantly feeding in and monitoring new information – at levels that would be difficult to maintain – to uphold safety levels, resulting in a level of protection that they would likely be unable to manage on their own.
A key feature of the tool is that it focuses on understanding customer use case profiles and expected behaviour, rather than a blunt set of static rules. Over time, this leads to fewer false positives as the system recognises patterns of fraudulent behaviour – resulting in a higher approval rate, more payments being completed, happier customers, and more revenue for the business.
In addition, fewer potential fraud alerts reduce back-office costs, while also leading to a reduction in manual reviews and lower numbers of frustrated customers asking for support.
While machine learning can dramatically improve fraud detection, AI isn’t going to replace human intervention entirely. There will always be a need for an element of human oversight because, while machine learning is very accurate and improving all the time, fraud detection also requires people who have the ability to review so-called edge cases (situations that only arise in a very unusual circumstances).
“There's always a team of people to review the outputs, because there's always outlier cases that may need to be manually reviewed,” said Nuruz Zaman, Senior Product Manager at Thredd. “Even with machine learning, it could never capture 100% of your fraud.”
Of course, deploying machine learning for any use case, including payment fraud detection, comes with its own set of unique challenges, such as the need to ensure businesses are using accurate data in their modelling.
In simple terms, AI machine learning is dependent of the quality of information it is evaluating. It requires enriched data to work effectively. This can range from how the cardholder authenticates themselves to the process they go through in the checkout journey, such as how many clicks and the number of pages they visit.
“You need a holistic view of the cardholder – 100% of the authorisations, all of the good customer behaviours, to feed into the model to show that this general activity is good,” said Gudgeon. “You also need accurate fraud labels – not just we think this is fraud, but we know something is fraud. That’s really important to differentiate against. If you think something is fraud, it’s open to interpretation. But if you know something is fraud there’s no ambiguity.”
So, with fraud rates escalating, criminals becoming more sophisticated and innovative technology being implemented by both sides, how can payment providers navigate their way through?
“As non-cash payments have exploded over the last 30 years, there is no question that ongoing risk management and fraud prevention will remain an essential component of managing any payments programme,” said Ava Kelly, our Chief Product Officer. “The inherent challenge is staying ahead of new scams while also providing a frictionless user experience. Given these high stakes, we believe that partnering with experts with a dedicated focus on evolving fraud detection will help keep savvy issuers ahead of the fraud curve and achieve their payment programme objectives.”
Get in touch
If you’re interested in adopting machine learning in your fraud prevention strategies and want to find out more, get in touch to speak to one of our experts.
Sources
1. PYMNTS
Sign up to receive Industry news, events and insights delivered straight to your inbox.